Congrats to our 2023 AICoE Seed Funding Awardees!
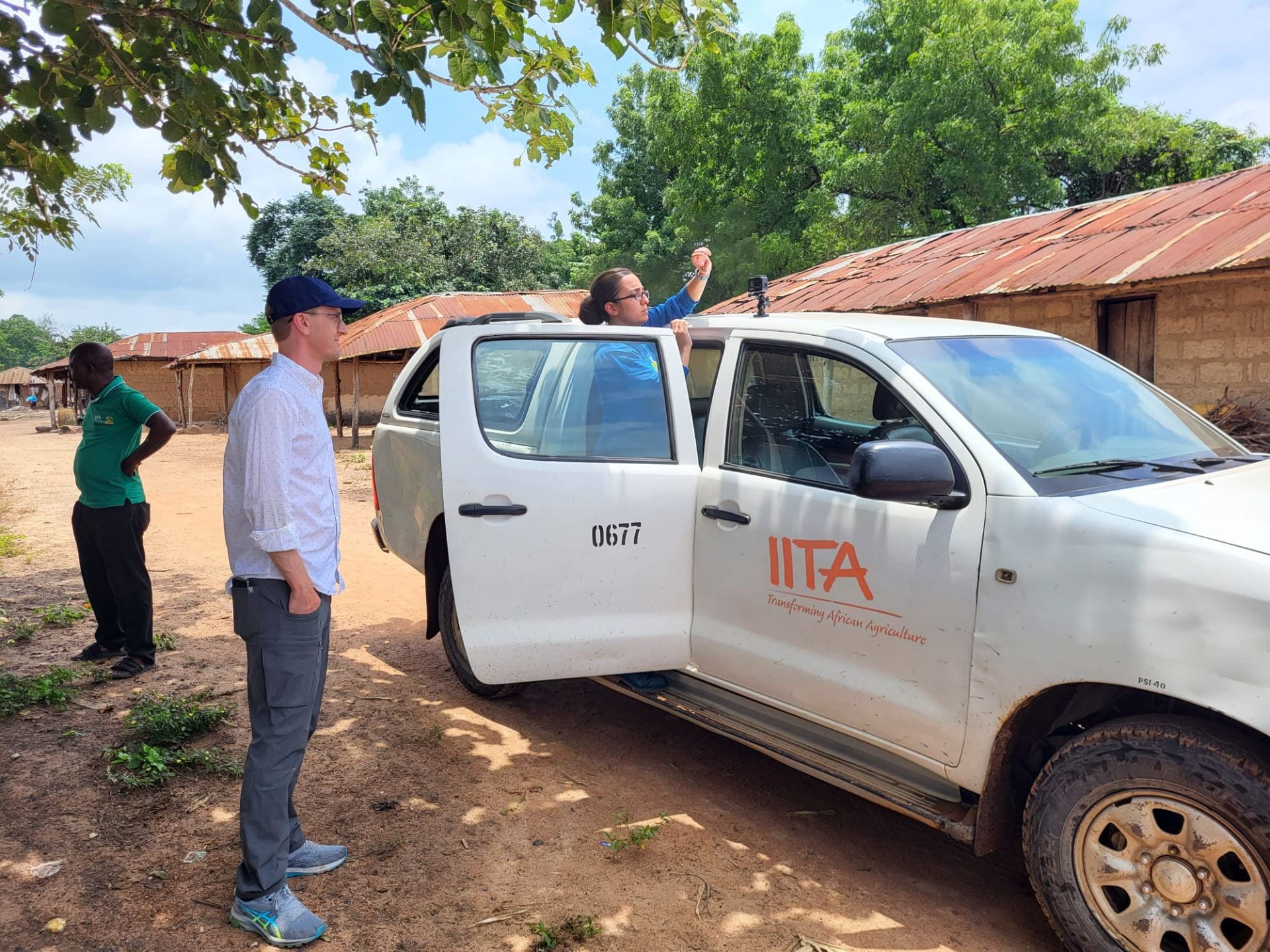
Flexible AI for Mapping Agriculture in Africa
PI: Kyle Davis (Department of Geography and Spatial Sciences):
Co-PI: Federica Bianco (Department of Physics and Astronomy)
Project Abstract: Agricultural data scarcity is a critical information problem that prevents many nations from developing sustainable agricultural interventions. The lack of reliable and regularly collected statistics limits an accurate picture of the state of agriculture and food security in many African countries and elsewhere. Using remote sensing and machine learning to estimate the extent of croplands and aquaculture across vast areas offers a novel alternative for gathering agricultural data and monitoring environmental conditions in places where large-scale agricultural censuses are logistically and politically infeasible. Using Nigeria as a case study, this research will develop transferable, scalable AI approaches – applicable to other data-scarce agricultural regions – to automate the delineation of crop fields and fish ponds across the entire country, contributing directly to food security and sustainability efforts in Africa’s most populous country.
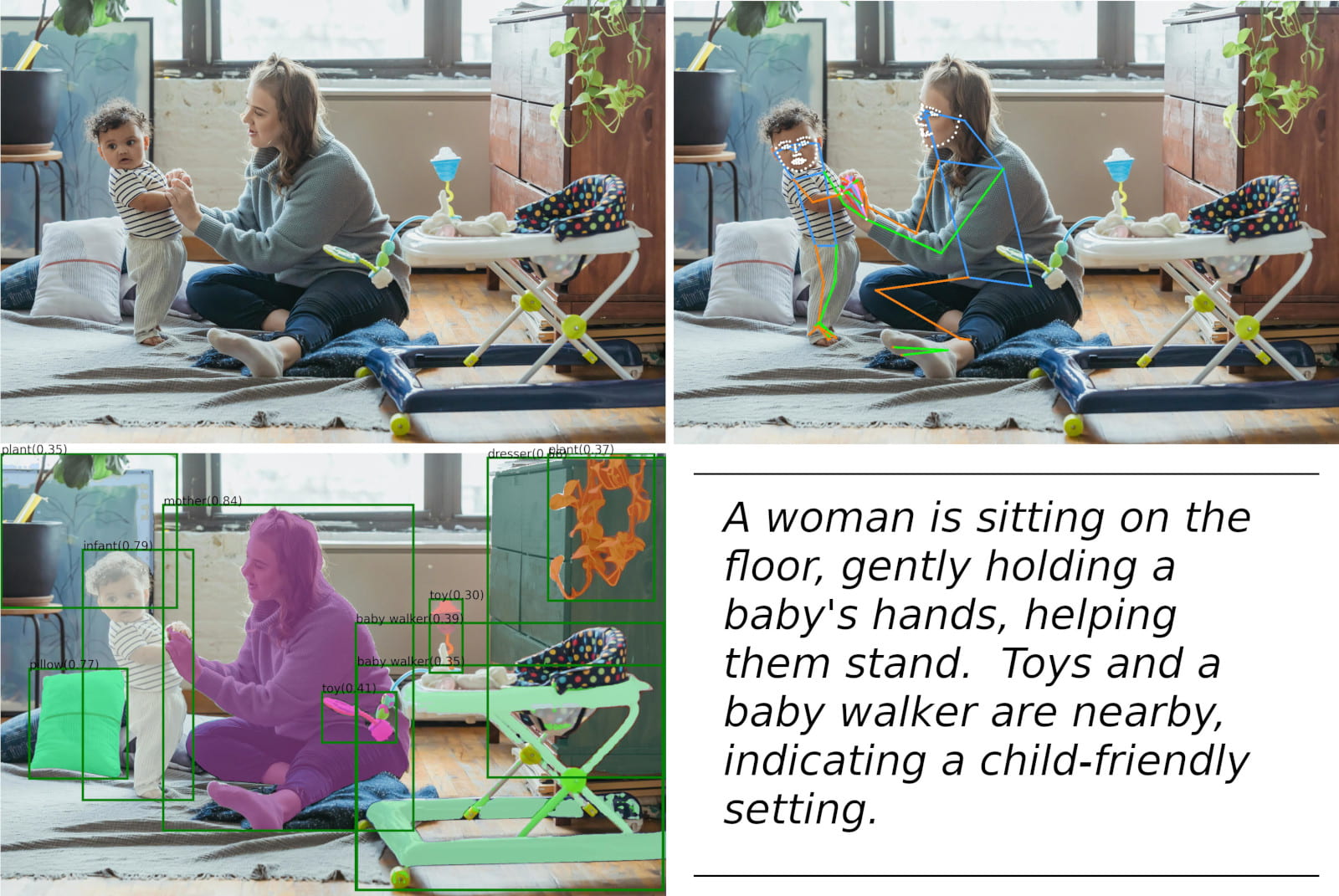
Longitudinal Video Analysis of In-Home Infant-Parent Interactions for Motor Skills Interventions
PI: Christopher Rasmussen (Department of Computer and Information Sciences)
Co-PI: Michele Lobo (Department of Physical Therapy)
Project Abstract: Enriched parent-infant play activities can positively impact motor, cognitive, and other developmental domains for both typically developing infants and those with or at risk of delays. To measure outcomes, current protocols require human observers to laboriously “code” body pose estimates, object interactions, environmental context, and high-level behaviors. This research aims to apply recent advances in neural network-based visual person tracking, object detection, and multimodal language models to streamline and enhance this process.
Such automatic, real-time video analysis may enable quicker detection of developmental delays, improve planning and monitoring of interventions, and even supply feedback to parents. Longer term, these techniques could also be applied to address aging in place issues like fall detection, memory assistance, and in-home stroke rehabilitation.
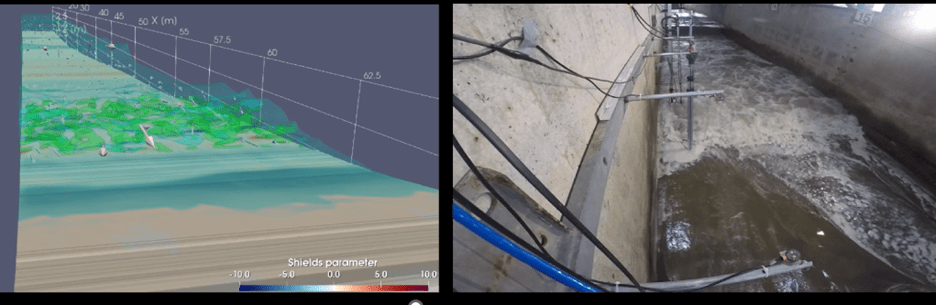
AI/ML Enabled Surrogate Modeling to Tackle Multi-Scale Challenges Due to Climate Change
PI: Tian-Jian Hsu (Tom) (Department of Civil and Environmental Engineering, Center for Applied Coastal Research)
Project Abstract: Climate change has resulted in sea-level rise and enhanced storm intensity that impact the coastal communities via flooding, land loss, and altered biogeochemical balance in coastal ecosystems. Conventional modeling tools tackling these regional-scale issues rely on highly empirical closures of surface waves, sediment transport and biogeochemical production/control that are no longer adequate for these extreme events. Conversely, many high-fidelity numerical models that are designed to resolve these small-scale processes are too computationally expensive to be coupled with regional-scale models. Our overarching goal is to use physics-informed AI/ML methods to revolutionize our capability to solve multi-scale modeling challenges in aquatic environments. For this seed project, we propose to create two AI/ML-enabled surrogate models for (1) flocculation dynamics (2) wave shoaling and breaking processes. Due to the importance of these two processes and the limitation of existing regression-based predictors, the creation of new AI/ML-enabled models is timely, and they will provide preliminary results for new proposals.

Leveraging Machine Learning and Nanocomposites-based Wearable Sensors to Analyze Human Movements and Detect Abnormalities
PI: Sagar Doshi (Center for Composite Materials)
Co-PI: Matthew Mauriello (Department of Computer and Information Sciences), Jill Higginson (Department of Biomedical and Mechanical Engineering), Erik Thostenson (Department of Mechanical and Materials Science and Engineering)
Project Abstract: This seed grant aims to leverage machine learning models to transform nanocomposite wearable sensor data into insightful information for biomechanics researchers/clinicians – streamline data processing, identify gait patterns, detect abnormalities, and visualize results in an easy-to-interpret manner. This study will establish a collaboration between CoE’s three fast-growing research centers – Center for Composite Materials (CCM), Engineering Driven Health (EDH), and AICoE to combine materials innovation and machine learning models focused on improving patient outcomes. The project team has developed and patented novel carbon nanocomposite sensors that are thin and flexible and enable measuring human gait and movements outside a laboratory. The key objective is to adapt/extend off-the-shelf Machine Learning techniques to analyze data collected from our wearable sensors to evaluate critical gait parameters such as applied load, walking surfaces (flat, up/down the stairs), walking speed, heel strike, and other abnormal gait events.
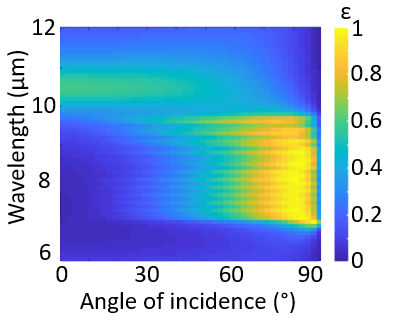
Adaptive Thermal Emission Design Driven by AI
PI: Xi Wang (Department of Materials Science and Engineering)
Co-PI: Austin Brockmeier (Department of Electrical and Computer Engineering)
Project Abstract: Controlling the thermal emission properties of materials has applications in heat management, radiative cooling, and solar heating, where benefits can range from reducing carbon emissions for more environmentally sustainable technology to improving thermophotovoltaic systems. Materials with specific thermal emission properties can be created through the precise arrangement of nanostructured materials. However, current methods for designing materials to achieve control of thermal emission still heavily rely on experience and iterative, time-consuming numerical simulations on a case-by-case basis as there is no general closed-form solutions. Therefore, for this seed project, we will use data-driven AI methods, creating a comprehensive model that can be used to optimize the design of materials with thermal emission control. This study will promote a deeper understanding of emission properties and identify designs that have the potential to achieve precise thermal emission properties.
AICoE Seed Grant Awardees 2022
The following projects were funded in our first round call for seed funding.
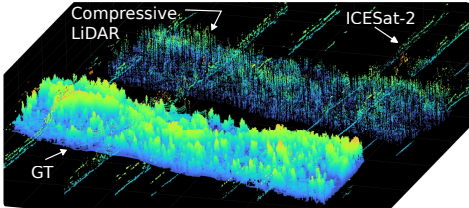
Machine Learning for Computational LiDAR Imaging in Earth Science
PI: Gonzalo Arce (Department of Electrical and Computer Engineering):
Co-PI: Rodrigo Vargas (Department of Plant and Soil Sciences)
Project Abstract: The Earth is continuously changing due to both natural processes and the impacts of humans on their environment. Observing and understanding our planet in order to predict future behavior as reliably as possible is of great importance today and for the foreseeable future. It will help manage resources, mitigate threats from natural and human-induced environmental change, and to capitalize on social, economic, security opportunities. Among the many tools available in remote sensing, light detection and ranging (LiDAR) imaging is an unmatched technology that provides 3D sensing for Earth science.
This research aims to advance and innovate LiDAR satellite sensing as well as translate these advances towards beneficial societal outcomes. This collaborative work engages scientists & engineers across the Colleges of Engineering and Agriculture and Natural Resources

Data-driven Explanations for Knowledge Discovery in Seabed Morphodynamics Analysis
PI: Xi Peng (Department of Computer and Information Sciences)
Co-PI: Arthur Trembanis (Department of Marine Science and Policy)
Project Abstract: Many naval applications require the ability to consistently and reliably solve large-scale machine learning (ML) problems on local, regional, and global scales. Examples include seabed morphodynamics and acoustic imagery analysis. Such applications often collect large-scale geo-distributed data at unprecedented resolution. Making use of this data to gain scientific insights into complex phenomena requires characterizing dynamics and uncertainty among a large number of variables. Characterizing by large non-stationary distributions, such data poses an unprecedented out-of-distribution (OOD) challenge where ML models often operate on unseen distributions due to diverse noise sources, unknown uncertainty levels, and long-range spatiotemporal dependence.
This research departs from conventional spatiotemporal – or time and space – modeling to develop a first-of-its-kind OOD-resilient methods for explainable seabed morphodynamics analysis. The OOD-resilient data-driven explanations will enforce scientific consistency and plausibility to deepen understanding or reveal discoveries that were not known before.

Radical Fashion: Digital Historical Reconstruction of 1920’s Garments for Virtual Exhibition
PI: Kelly Cobb (Department of Fashion and Apparel Studies)
Co-PI: Dilia Lopez-Gydosh (Department of Fashion and Apparel Studies)
Co-PI: Belinda Orzada (Department of Fashion and Apparel Studies)
Project Abstract: Museum exhibits transform scholarship from private act to a public experience because they reach a wider audience than most journal articles and referred presentations. The Department of Fashion and Apparel Studies is developing a historic fashion exhibition “The Twenties”, where the researchers will develop a digital, historical reconstruction of 1920’s garments housed in a virtual gallery. The virtual gallery will feature selected digital garments that are too fragile to be displayed on mannequins.
The research team will build digital assets including digital textiles and texture maps, embellishments applying TEXTURA – a cloud native AI technology by SEDDI, who is an industry partner of the team. Digitally reconstructing fragile garments allow viewers to experience the drape, silhouette and textile details on the body and in movement – something that garments exhibited on a mannequin or laid flat are unable to communicate.

Transformers for Socioemotional Behavior Assessment of Children with Autism
PI: Leila Barmaki (Department of Computer and Information Sciences)
Co-PI: Anjana Bhat (Department of Physical Therapy)
Co-PI: Kenneth Barner (Department of Electrical and Computer Engineering)
Project Abstract: The number of children diagnosed with Autism Spectrum Disorder (ASD) has increased in the past few years. Children with ASD have significant social-cognitive impairments in imitation, motor planning, emotional connections, as well as primary motor impairments in posture, balance, gait and coordination.
This research will use AI and deep learning methods for further advancement of the understanding of socioemotional behaviors in children with ASD. The aim is to eventually integrate existing models into a data collection pipeline to collect and analyze socioemotional behaviors of the children almost in real-time.
Predicting After-effects of ExoSkeleton-assisted Gait Training to Inform Human-in-the-loop Control Optimization
PI: Fabrizio Sergi (Department of Biomedical and Mechanical Engineering)
Co-PI: Austin Brockmeier (Department of Electrical and Computer Engineering)
Project Abstract: Robot-assisted rehabilitation is a promising intervention for supporting recovery after neuromotor injuries. In this context, robotic devices such as wearable powered exoskeletons are used to physically interact with the participants during their movements. Training is usually provided to target changes in neuromotor coordination that will translate into improvement in walking function measurable after completion of intervention. Currently, there is a limited basis to form a prediction of how effects measurable during training will translate into changes in coordination after training.
The aim is to identify the relationship between features of propulsion mechanics measured during training and the features of propulsion mechanics after training. Being able to predict the after-effect will enable real-time adjustment of the exoskeleton control to achieve desired outcomes.